
The topic of "Introducing Statistics" made me think of a very exciting opportunity I had the chance to be a part of this semester. Since January, I have been involved in my first teaching assistanceship at Emory University. I am an instructor of a hypothesis-driven introductory laboratory course for undergraduates. While many of the students are familiar with many of the basic lab techniques - pipetting, sterile procedure, plating bacteria - this was the first time that they were exposed to one of the classic statistical tests: chi-squared. And now, I was the person to introduce this crucial facet of statistics to them - talk about an important first introduction.
The lab period was designed to ease the students into using the test. We used a particularly good module from Math Bench as our learning tool, which explains the roles of observed and expected values in the calculation, the relevance of degrees of freedom, the importance of p-values, and how to determine significance from their results.
I enjoyed guiding them through the process and answering their questions - the biostatistics course served me well.
Once our initial foray into the realm of statistics was over, I was pleased to find that many of the students took to the lesson quite well. Several engaged me in a more detailed discussion of degrees of freedom during the next class, and all were eager for advice on how best to incorporate statistics into their final projects.
Unfortunately, it was during the revision process of these final projects that I truly had the chance to see what lessons my students had taken to heart. The situation I found myself in is illustrated perfectly by this poignant (if lengthy) comic by Randall Munroe, the brilliant scientific cartoonist behind the webcomic xkcd.
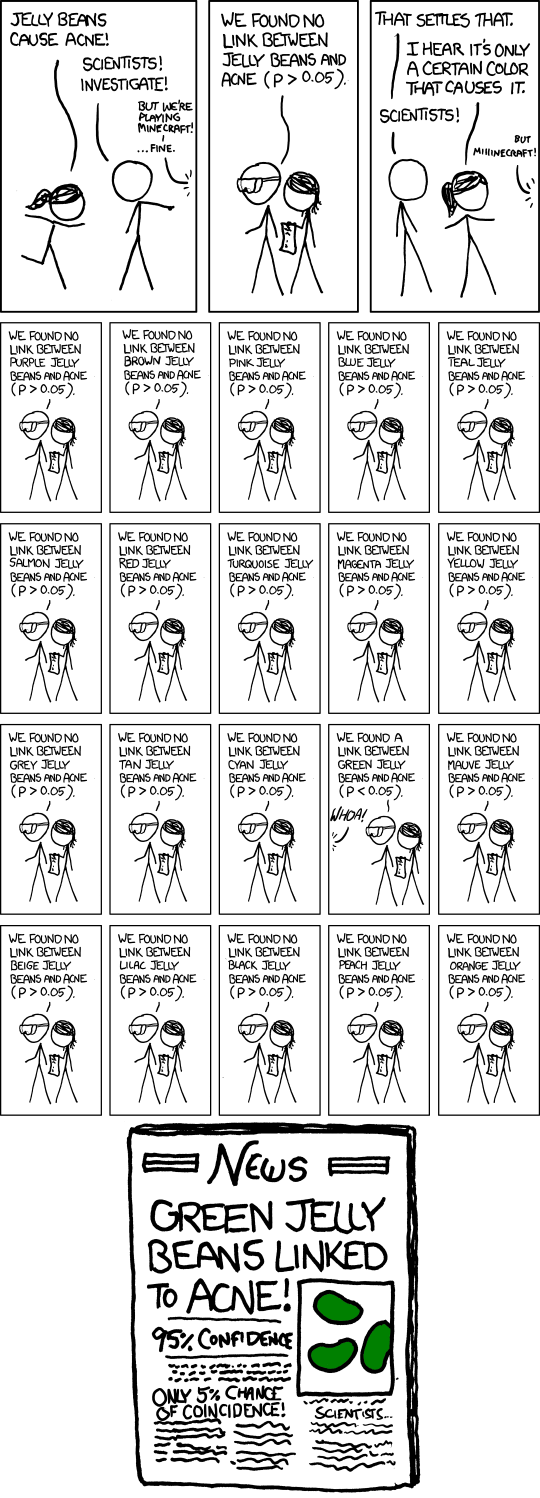
Here, my students play the role of both the excited news journalist and the beleaguered scientists, diligently carrying out their experiments until the moment they are free to jump to disproportionate conclusions. While my students know how to use the chi-squared test, they lack a deeper understanding of its applications, and are prone to taking its output as gospel. Any significance they find, no matter how slim, is certainly newsworthy, and sufficient to state that they have answered all the remaining research questions in the field of biology.
Upon reading their extravagant claims in their final projects, I was surprised. But upon reflection, it got me thinking about how I, as a student in statistics, would be seen in their position.
Just like my undergraduate students, I am prone to behaving like a child with a shiny new toy. Granted, my toys - paired t-tests, two-way ANOVA - might be a bit more complex than the ones they are working with, and might require a little more know-how and assembly to get them up and running. Nonetheless, here at the beginning stages of my statistical understanding, I find myself tending to default to trusting the output of a test run in Prism, even if I am not completely clear on what exactly that output means.
In this way, I am no better off than a reporter excited about jelly beans.
While I might be the instructor, the absolute confidence of my students in their chi-squared results opened my eyes to a very important lesson. It is never enough to simply trust in the power of statistics, as awesome as that power may be. Without a complete understanding of the tests you are using, you are destined to abuse them.
What an enlightening experience! I do think it is unlikely for a non-statistician to pursue and attain a “complete” understanding of various statistical tests. We all just have different things to do since our specialties lie elsewhere, and for the students among us, our statistics courses are designed with that in mind. I was just thinking of my readings from the course textbook Intuitive Biostatistics by Harvey Motulsky and how I’ve benefitted much in terms of being able to think of and categorize different statistical tests. But when I considered having a complete understanding, I asked a question I hadn’t thought of before: I don’t possess a strong command of the mathematical computation that underlies some of the statistical tests we’ve learned about; why didn’t Harvey delve into that? I pulled out my book and read the cover. The subtitle: A Nonmathematical Guide to Statistical Thinking. And “nonmathematical” is in bold font – so that we biologists flock to it, I guess! All of us who are not statisticians may never attain that full understanding of the tests we use. We may learn the concepts and mathematics, and I think the mathematics are the first thing out the window. But using resources like this book and this course, as long as we retain a firm understanding of the concepts behind the tests, we can minimize or even eliminate our abuse.
ReplyDeleteKelsey, I can relate to this 100%. As an undergraduate student myself who has taken the introductory lab course you speak of, I can fully attest to the concept of an "ooh-aah" moment in reaching some sort of statistical conclusion, albeit one that holds little if any validity. I often find myself, along with my peers, trusting traditional statistical methods without truly questioning their relevance to the specific data sets at hand. When I was writing my thesis, I found that I resorted to analyzing all my data with either a Mann-Whitney U test or a Spearman's correlation, mostly because those were the tests most used in my lab's publications. It wasn't until I was deep into the curriculum of this course when I started to think independently of the published status quo and choose a statistical method that best fit my data sets. During browsing for the BadStats assignment, it became abundantly clear that a lot of researchers in the field fall prey to this short-sighted nature of statistical analysis. For example, I came across so many papers wherein multiple t-tests were used, rather than an ANOVA, thereby increasing type 1 error. Had the authors thought about the repercussions of each method used rather than taking the stats yield as face value, an error like such would likely have not been made. Overall, I think you make a fine point that the power of statistics is futile when those using it accept outputs as dogma.
ReplyDeleteI enjoyed this post and seeing how you were able to learn from your students! I believe this has happened to all of us (and keeps happening to more senior researchers, as were able to see in the Bad Stats assignment), given it is fairly easy to get carried away in the assumptions that we have made a major breakthrough and we’ll have a great impact in our field. However, it is as you said, the key lies in the proper understanding of the different statistical tests and our interpretations of the results. As we are beginning to use different statistical analyses in our research, we sometimes rely on others with more experience to instruct us as to what test to use, given our experimental design. The sad part is that many people have not taken a biostatistics course like this one, where they teach us when it is more appropriate to use a specific test. So here starts our confusion about concepts like “why it is wrong to use multiple t-tests instead of an ANOVA?” and not understanding that this leads us to “p hacking”, sometimes without us even noticing. It is because of this that the job you are doing is a very important one, given you are the first person to have an impact on the way they approach data analysis and the importance they give to it. The best way to combat the confusion so many researchers have about the correct usage and analysis of statistical tests is by education.
ReplyDeleteKelsey,
ReplyDeleteI liked your post for several reasons. First, who can resist a shiny new toy? Great title!
Second, I taught the Emory undergrad biolabs many times and struggled mightily trying to teach the math, logic, and interpretation behind using some statistical test to analyze data. I don't pretend to have any great insight here, but I think getting the students to think about objective analysis of their quantitative data is probably the biggest teaching point. Whether they use the test appropriately or draw the proper conclusions (or use the correct language to communicate the results of their statistical test, which is rare) is really secondary. So I would not sweat the undue emphasis the students place on a p < 0.05 from an under-powered design. At least the labs got them thinking about tests to analyze their data. I don’t expect my infant to walk across the room even though he can support his own weight standing and can move his legs. He’ll figure out walking with practice. Same thing applies for your students (and you and me).
Which brings me to my third reason for liking your post, in our own work and in teaching good practices to our students; we should recognize something Dr. Murphy stressed: consulting a statistician early in study design. If anything, Dr. Murphy may have underemphasized this. Only one of my past mentors/labs (out of 7) actually practiced this and impressed upon me the near necessity of doing so. Other labs were either set in their ways, know-it-alls, clueless, or just didn’t see it as a priority. All labs are faced with garbage in, garbage out, but I suspect the labs that don’t consult statisticians have more garbage.
Fourth, “teaching and learning”: That is kind of a buzz phrase in education parlance. I was never totally sure what it meant, but I think you lived it. The teacher doesn’t just teach, and the students don’t just learn. It goes both ways. By observing your students’ overzealous interpretation of a significant chi squared test and reflecting on your shiny new tools, I think you benefited (learned).
Good luck with your research and teaching!